Federated Learning (FL) enables collaborative AI model training across edge devices while preserving data privacy — a critical need for IoT and mobile systems. Yet deploying FL at scale introduces challenges: heterogeneous data/resources, dynamic device availability, adversarial attacks, and cross-platform integration. This tutorial bridges theory and practice, offering a systematic framework for designing robust FL systems at the network edge. Attendees will gain insights into convergence guarantees under arbitrary client participation, game-theoretic incentive mechanisms, resource-efficient deployment strategies, and defenses against data poisoning. Case studies and demos (e.g., cross-platform FL for Android/iOS, privacy-preserving campus analytics) will illustrate deployment best practices. Designed for researchers and practitioners, this tutorial aligns with ICDCS2025’s focus on the theory, experimentation, and practice of Distributed Computing Systems and Distributed Artificial Intelligence. Participants will leave equipped to tackle system heterogeneity, resource constraints, mechanism design, and adversarial threats, real-word deployments for FL systems at the future network edge.
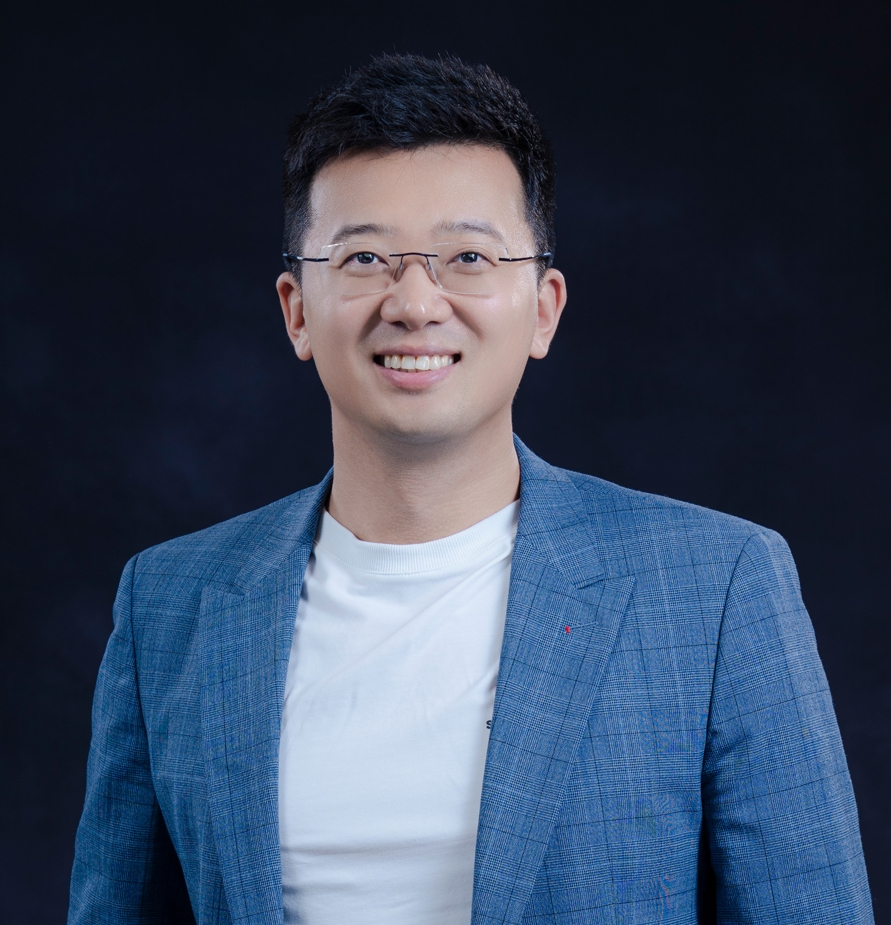
Bing Luo
Duke Kunshan University
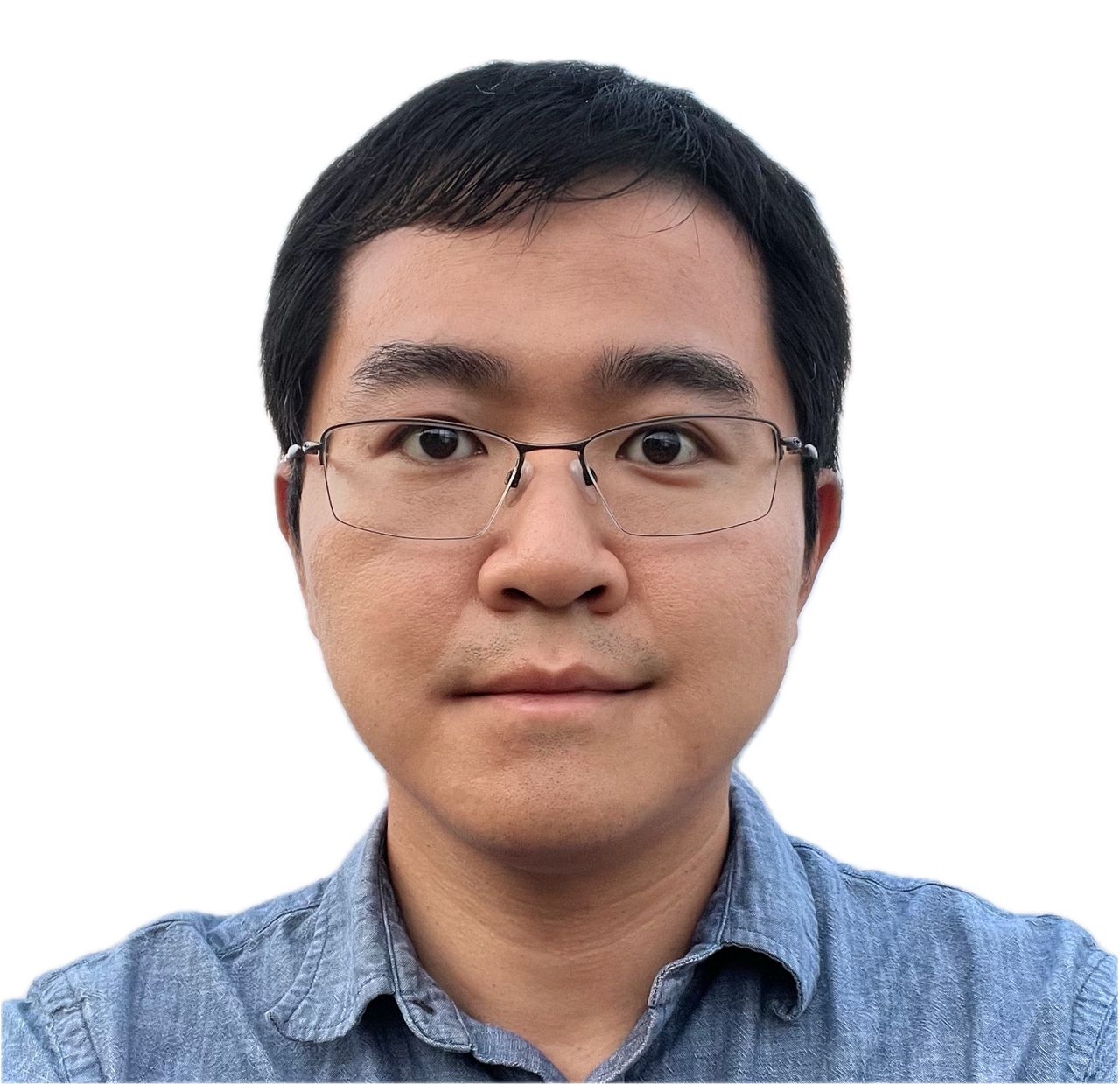
Shiqiang Wang
IBM T. J. Watson Research Center
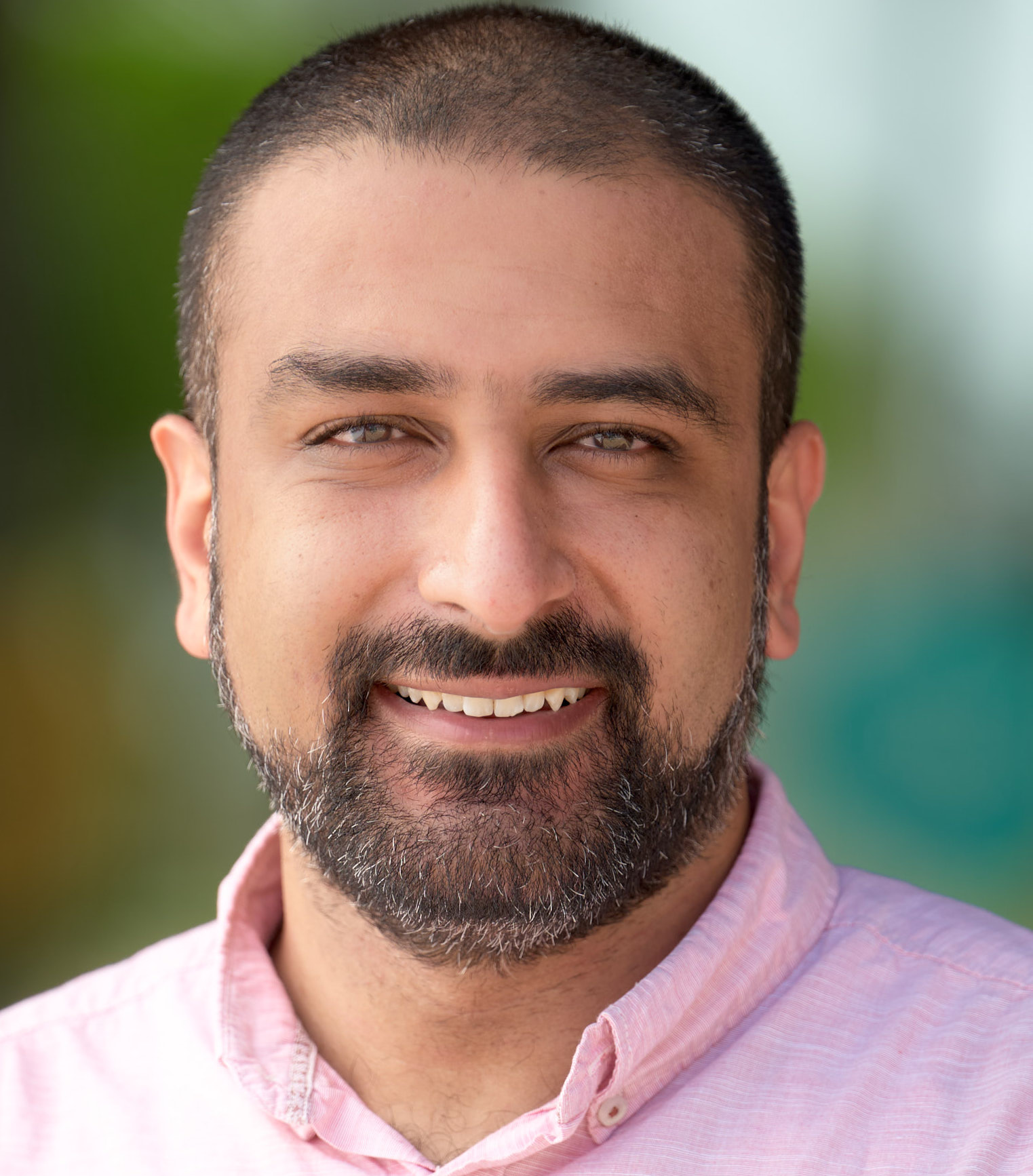
Ahmed M. A. Sayed
Queen Mary University of London